How to Create a Business Model of a Loyalty Program
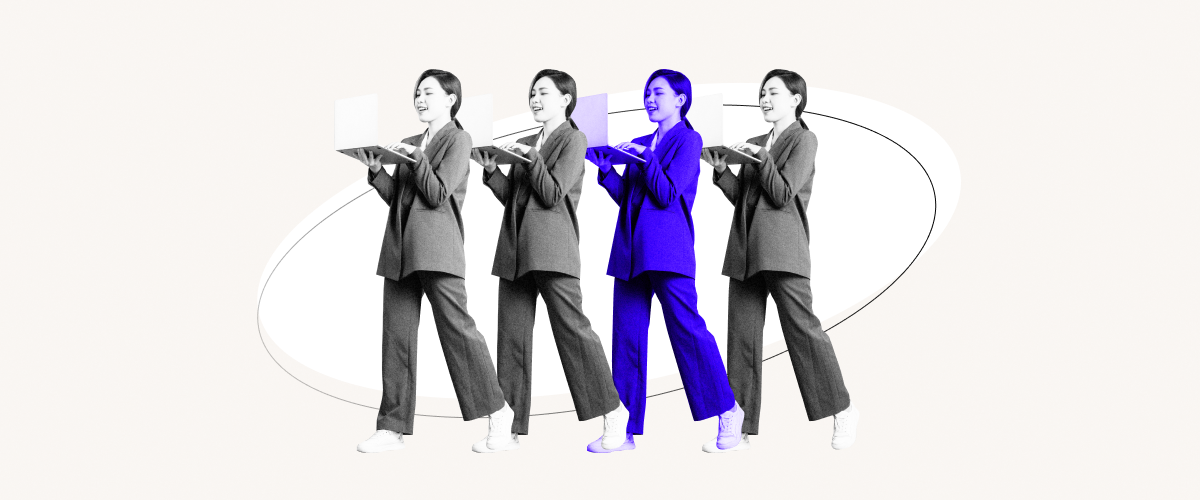
How to create a loyalty program model that would help you increase sales? This case study demonstrates how customer analysis, proper data segmentation, and an effective loyalty program model can help a brand increase revenue and foster customer loyalty.
When you see the real numbers, it is easier to get an objective view of a business and see the dynamics in marketing and sales. Thus, a financial model of a loyalty program can help you achieve the business goals. We calculated this model using an Excel table and now want to share the results.
Our analysts use Excel tables to create loyalty program models. You can download a free financial model template in Google Sheets below and build a model on your own.
One can use different loyalty program models depending on the type of business one’s dealing with.
Each loyalty program model can be profitable, but if you want to find out which one is perfect for your business, you need to analyze several factors:
In the case study below, we’ll share our financial model of a multilevel loyalty program. For this loyalty program model, we needed to calculate:
Our first step was to gather all of the data on the transactions and customers' interactions with the brand.
The purpose of a loyalty program is to motivate customers to buy more: e.g., without a loyalty program they would spend $50, but thanks to the loyalty program, they might spend $65. However, sometimes it’s not really clear why customers spend more: whether the reason is the loyalty program or the customers are just loyal to the brand.
That’s why our financial analysts should create two forecasts:
This kind of analysis requires retrospective data: we should analyze how the sales are going right now, when the loyalty program has not been launched yet.
Customer analysis plays a crucial role in building a loyalty program model: it's important to understand how loyal your customers are at the initial stage.
When we collected and processed the data, we got the following useful info:
We also created a graph to take a closer look at the clients and see how often they bought something.
The next step was to analyze the loyal customers: we analyzed the number of purchases and the time period between them.
Another method of dividing customers and analyzing their behavior & loyalty is RFM analysis. We made an article on how to conduct this analysis.
This forecast answered the following question: “What will be the sales dynamics if we leave the situation as is?”. In our case, offline sales were growing and online sales were dropping. We needed to analyze the current situation considering the following factors:
Using data segmentation, we forecasted what sales we would get without the loyalty program.
You can’t create a financial model of a loyalty program being 100% sure that your sales will grow; instead, look at the real numbers and don’t forget about previous trends and seasonal activities.
As we’ve mentioned before, we picked a multilevel loyalty program model: the discount would grow depending on the purchase value. We created four variants of this loyalty program model.
However, you can’t just assume that sales will grow and your stores will be crowded. That’s why we suggested three forecasts for each loyalty program model: pessimistic, conservative, and optimistic.
Thus, we created four loyalty program models in three versions (optimistic, conservative, and pessimistic) for offline and online.
Still, we needed to consider the number of new clients to come. We didn't know the exact growth, but we could create a forecast. To make our predictions more accurate, we suggested three of them: pessimistic, conservative, and optimistic.
However, the growth might depend on the discounts too: a bigger discount could attract more clients. Thus, different business models suggested different growth:
We’ll showcase the examples for the online loyalty program model only.
At this point, we have the data on clients and purchases and a loyalty program model. The next step is to bring together the data and the loyalty program.
In our case, we gathered the existing data on the clients and their purchase values and divided them by their status in the loyalty program. Thus, we understood how many people would get this or that loyalty status.
Some customers were close to getting a discount. For example, if the loyalty status began with $150 spent, customers who’d spent $130 were easy to convert into a new loyalty level.
This way, we segmented the customers according to their loyalty status. The more the client bought, the more loyal they were considered.
The final part is to calculate the sales uplift brought by the loyalty program. At this step, you need to consider the following points:
To choose the model that will increase your sales, you need to create monthly forecasts for the loyalty program models using the factors we’ve mentioned in the previous section. Each case is unique, but you may use our example and see the way we organized the data in the Excel table below.
First, let’s sum up how we calculated our loyalty program model. We created:
As a result, we used 312 Excel tables for all the calculations.
The next step was to turn the monthly data into a yearly forecast. Thus, we put together the figures and created a forecast for a year in three versions: pessimistic, conservative, and optimistic. After that, we compared this forecast with the previous forecast without the loyalty program.
In this table, we put together online and offline sales.
The final step is to choose the most efficient loyalty program model: the one that gives you the bigger uplift with lesser expenses on rewards.
In our case, we got the following winner: a loyalty program model that offered 0%, 10%, 15%, and 20% discounts for $100, $200, 370, and > $370 purchases.
In this case study, we described only one way you can calculate a certain loyalty program model. Although you may face other issues with your unique loyalty program, the principles are pretty much universal: